Sunday, January 22, 2023
The Future Of Online Media - How Generative AI Fundamentally Changes The Landscape
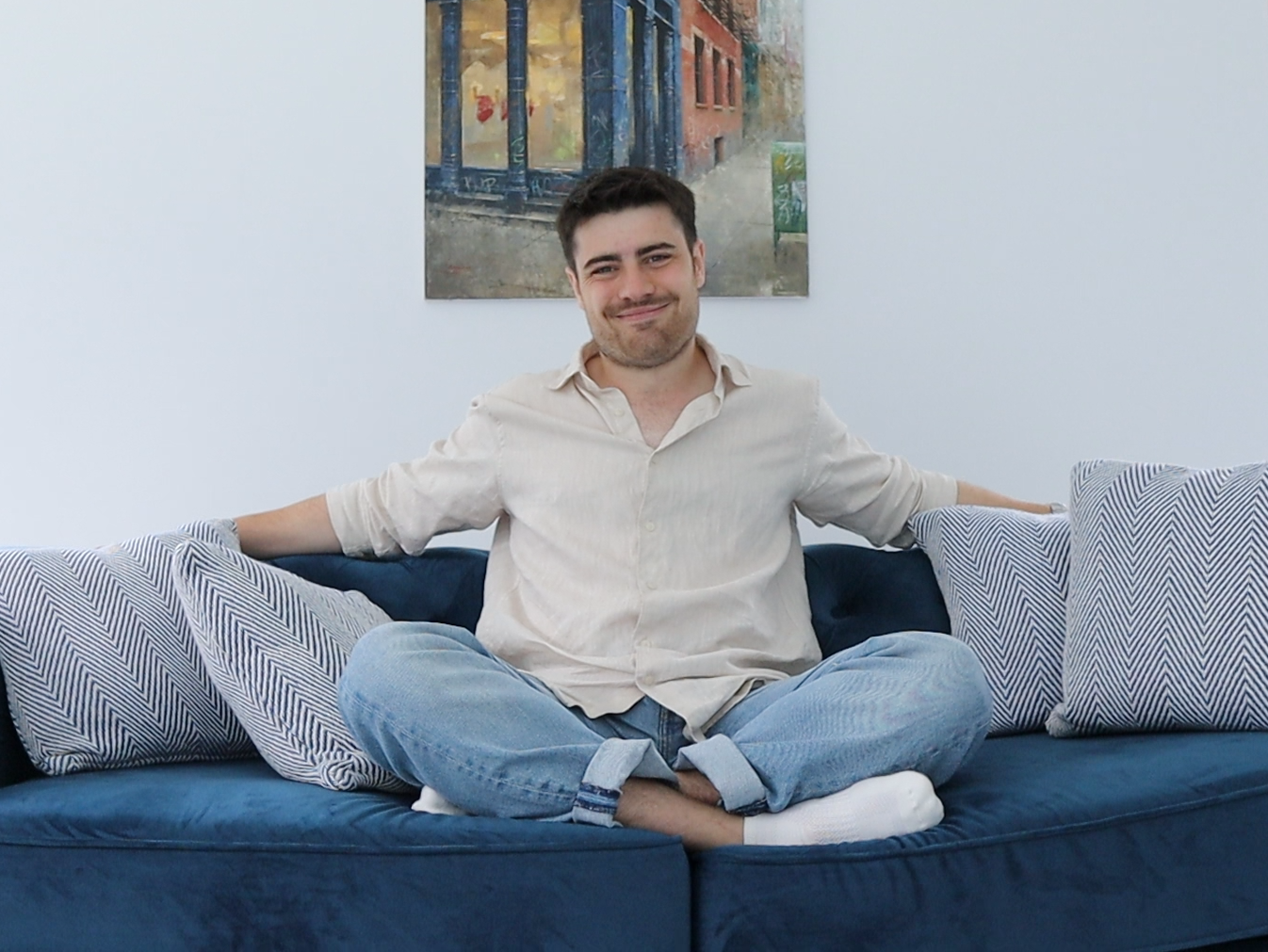
Charlie Sweeting
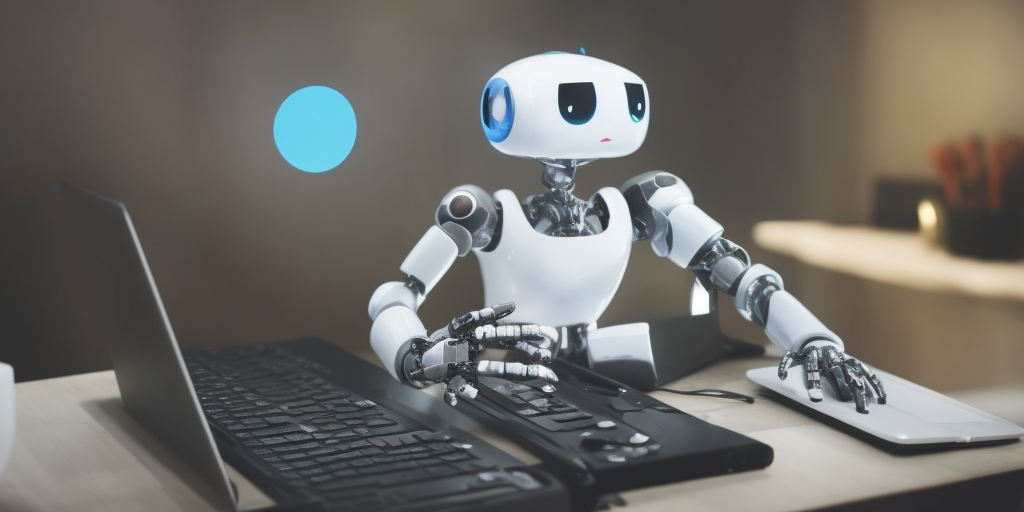
Ideation, creation and distribution. That’s the flywheel that every piece of media past, present and future goes through before it reaches you. Doing all three well generates attention, doing them frequently maximises it and doing them cheaply optimises the return that you make when monetising it.
Each iteration represents a learning opportunity, a time to understand whether your idea resonated within the current zeitgeist and gain the information required to change your future approach to something which better reflects the needs of your audience. Over time, technology has improved every aspect of the flywheel and the iteration time has progressively quickened:
- The printing press, radio and television made the mediums of text, audio and video widely available - with the internet supercharging their distribution.
- Mobile phones made capturing audio and visual content trivial with personal computing lowering the barriers to editing it - democratising content creation.
- Real time analytics helped content creators understand their audience, showing what they resonate with, allowing them to create content in line with what interests viewers.
In combination, these technologies have given us our current media ecosystem. Widely accessible, massively tuned into the public consciousness and rapidly responsive - with creation measured in minutes for news and days for entertainment.
The latest advancement in our media cycles and entertainment generation is generative AI. Through providing a written prompt you can create long form text, audio, images and video without possessing the skills necessary to construct them from scratch - effectively making every creative choice an editorial one. It’s widely accepted that generative models will lower the skill requirement to create content even further. Which, on the surface, seems like the same kind of incremental improvement that we’ve historically seen when new technologies speed up a stage of the media creation flywheel. However, generative models have the potential to deliver a more fundamental step change in our global media ecosystem.
Until now there has been a non-trivial cost to creating online media. It’s lowered substantially in past years, but we are still limited by the constraints of time and money to create a relatively small amount of content. Despite those data limitations, humans have managed pretty well. We’re generally quite good at applying the context of what we’ve experienced over the course of our lives to a small number of relevant samples and extrapolating out learnings to the next batch of content that we create. This is something that algorithms are generally poor at.
That dynamic flips when the number of relevant samples becomes much larger, something which becomes very possible with generative models drastically lowering the effort requirement for content creation. When you cross that threshold you remove the most time consuming link in the content creation flywheel, us. You no longer need people to interpret the next step, you can use algorithmic models to predict what should be created next, learning directly from the analytics that we’d otherwise interpret. These models have a much larger working memory than us, predict much quicker than us, can consume a larger number of signals than us (if the dataset sizes are sufficiently large) and all together learn quicker than any human can. Moving from a human with editorial control to a model shifts the pace of learning to be bottlenecked from the pace of content creation, to the speed of content consumption. That change isn’t the incremental improvement we’ve seen historically with new technologies affecting the media landscape, it’s a fundamentally new one.
So what does this mean for us?
What does it mean when the cost of creating content goes to zero, the speed at which it can be created is near instantaneous and the speed at which it adapts to our tastes is several orders of magnitude higher than we’ve ever experienced?
Well, we’ve seen some analogous situations before.
The industrial revolution made it extremely cheap to produce many of the physical products that we consume which would otherwise be expensive to produce by hand. An economist might tell you that from this point forward there was never once a pot or pan made by hand again. What’s the point of paying many times more for something that has the same utility? A historian would tell you that this wasn’t the case.
We’re human.
We value some items in a utilitarian context, in so far as what they do for us, but we still value a soul. We attribute a higher value to things which are handmade and represent a much deeper human narrative. We care about who made things and why, we care about their heritage and what those things express to others about us when we buy them. So much so that we pay considerably more for them.
In the same vein, we’re likely to value media generated by models in a utilitarian context, such as instructions on how to put up a shelf, but we’re still likely to value media created by people too. This is a hard space for artificial intelligence to encroach upon. Even if models make something feel like it’s created by a human, so much so that it can convincingly dupe an audience, the very reveal that it wasn’t successfully destroys that illusion.
What doesn’t map well from the industrial revolution is the personalisation at scale that generative AI brings. Physical goods can’t quite be tailored to the individual at the same speed and low-cost as digital ones. So what happens when it’s economical to tailor content to the individual?
I think that evolution will reflect the same sensitivity to the divide between utility and art. We’ll embrace personalisation where it benefits us practically, but reject it where we want to feel part of something larger than ourselves. This basis of human connection that people can provide through their art will insulate a class of artisans against the efficiency of the models that we create - with both kept in a healthy equilibrium that reflects our practical and emotional needs as a society.
So far everything seems rosy. Machine learning is likely to take over the heavy lifting of ideating and creating media that has some practical nature to it, and humans can focus on the niche of media creation that has an emotional backbone that users can connect with. However, we’re presuming we can accurately communicate our full intent to the models we train.
That often isn’t the case. Take a model that is designed to create content which optimises for our attention. That model is agnostic to concepts like truthfulness and how “appropriate” the output is for a given audience. Those side effects open the door for disastrous consequences. We might not care that a generated cartoon is fiction, but our news sources reporting a nuclear explosion that never occurred would create huge societal fallout that is undesirable no matter how much engagement it generated. Humans naturally moderate these effects, we have a reputation that suffers when we’re untruthful which dissuades us from publishing easily falsifiable information. Navigating this barrier for models is much harder. It requires both that we can come to consensus on what that reward function looks like and that we can quantify it in a way that reflects our underlying intentions.
These kinds of problem aren’t new, there is already a line being carefully trodden with disinformation, clickbait and morality in media today. However, the further lowering of the barrier to creating believable content of infinite variety is going to make the questions of what is real and what should be consumed even more pertinent.
So, if we know the transformative nature that these models will have on our ecosystem, what that is likely to look like and the types of problems we’re likely to encounter - who are the likely stewards of that progress?
The natural answer here is those companies that have media recommendation models at the core of their current business.
There’s an affinity between media recommenders and generative models because, under the hood, they’re a lot alike. In fact, you can think of generative models as providing a more accurate response in the same feature space. Given their similarity, the stage is set for the proprietary datasets that power most large recommender systems to feed the initial attempts at generative media models - overcoming their harsh cold starts. When you couple that with the resources necessary to build those models, in terms of both compute and engineering, it seems that participation may be limited to a small set of existing media companies.
This view is consistent with the prevailing narrative of how generative AI is likely to transform the tooling landscape. Namely, big tech will overcome the massive capital requirements to build highly generalised models, companies with existing proprietary datasets will tune those models to serve highly specific use cases, companies without useful proprietary data will seek to build moats by moving first with an un-tuned model that will generate property data over time and companies that are unable to build moats with their accrued data will compete with each other on the battlegrounds of brand, distribution and capital efficiency.
However, there are different moderating effects when thinking about ideation as opposed to creation. The aforementioned brand safety risk from imperfect reward functions is a problem that disproportionately affects existing brands who have something to lose. This opens up startups, who can afford to get it wrong, to take the reins.
Regardless of who builds this future, the value capture from building models that are directly tuned into the public consciousness, without moderation by an intermediary, promises to be immense and represents a fuller picture of the potential impact of generative models.